Its name is Heliograf and it works for the Washington Post.
Every year, Heliograf writes hundreds if not thousands of brief informational stories for the prestigious outlet. Here’s a passage recapping a DC-area high-school football game from September 2017:
“The Landon Bears shut out the visiting Whitman Vikings, 34-0, on Friday. Landon opened the game with a 90-yard kickoff return for a score by Jelani Machen. Landon added to their lead on John Geppert’s five-yard touchdown run. The first quarter came to a close with Landon leading, 14-0 …”
Heliograf is not alone. Bloomberg employs Cyborg. Forbes uses Berties. The Associated Press never bothered to give its artificial intelligence a name (that we know of), but it generates thousands of earning reports per quarter.
All of these AI platforms do one thing well: relay information and data.
But they’re not investigative reporters. They cannot connect dots to define the zeitgeist of a generation. They can’t interview people and use contextual dialogue and quotations to construct a rich story. They can’t capture the full complexity of the opioid crisis in the U.S.
Making meaning: The Achilles’ heel of the machine
Writing, visual storytelling and other content-creation mediums need more than information to resonate with the audience at a deeper level. Great content swirls with empathy, curiosity and counsel. There is so much meaning that lives between our modern mosaics of information, and bots still struggle to employ the fundamentally human attributes needed to get at it.
Sure, people have tried to teach applications to think outside of the box. One of the most entertaining examples is InspiroBot, a free online program that auto-generates inspirational quotes over soothing imagery. Sometimes the stuff it says vaguely resembles wisdom:

But a lot of what it produces is way out in left field:
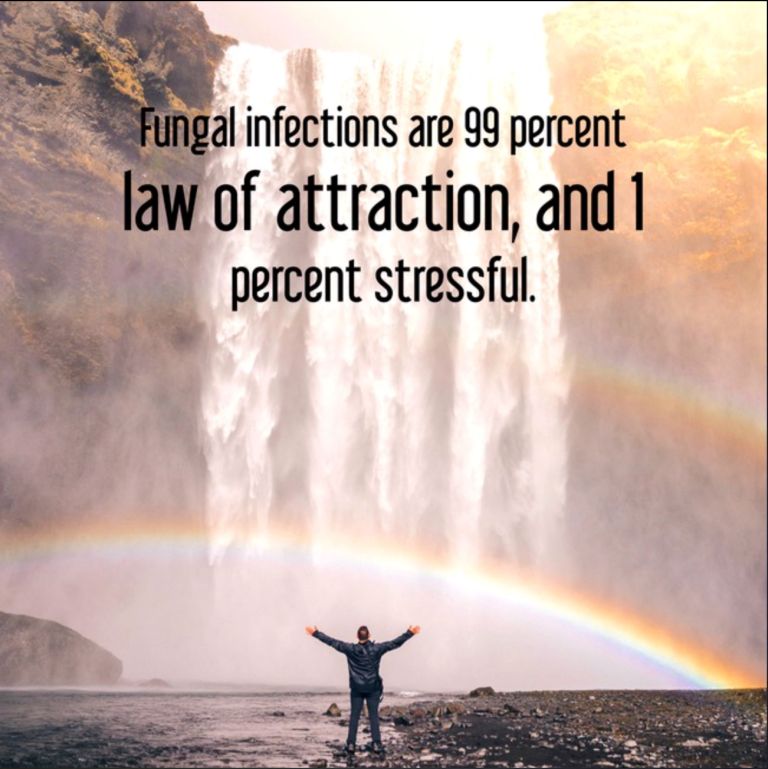
Or …

What using Apple products in bed has to do with rabies and power, I cannot say. But if these are darkly humorous, it certainly wasn’t on purpose.
We’re not 100 percent sure how InspiroBot works, because the creators of it haven’t told us. But a lot of its inspiration is nonsensical, sometimes dark, often humorous and entirely without any real meaning. These are randomly generated phrases presented in a discernible sentence structure, and any meaning brought to them comes from you, not from the messenger.
That word—meaning—is paramount. The intent to create meaning and the consciousness to knowingly convey it are non-existent in robots.
The intent to create meaning and the consciousness to knowingly convey it are non-existent in robots.
AI is, for all intents and purposes, completing very complex math problems to arrive at a statistical probability that two words make sense next to each other. Often, this is done in a certain situational context, like a chatbot or voice assistant responding to a query. And with machine learning, it can even improve over time.
But when it comes to creating relevant content that your target audience relates to on a human level, content creators of the homo sapien variety are very much in the driver’s seat.
Still, automated content creation certainly has its place among marketers, and it’s well worth exploring that role.
Taking a lesson from David Bowie
David Bowie exemplifies superb marketing, and believe it or not, is a curious example of using artificial intelligence as part of the content creation process.
For starters, you can call him a bit of a branding genius. From Ziggy Stardust to the Thin White Duke, the personas he created had a uniquely human brand of ingenuity, one that is perhaps every bit as memorable as the music he made.
I highly doubt machine learning algorithms could envision Bowie’s menagerie of personas, let alone construct their backstories and anecdotes that he mused about in song.
However, one might say that Bowie had an InspiroBot of his own, first in the form of the cut-up technique.*
In the ‘70s, Bowie would write out songs on paper, physically cut it up into individual words, and then put them back together again in a different order (Kurt Cobain and Thom Yorke would also use this technique for several songs).
In the ‘90s, Bowie developed a digitized version of the cut-up technique called the Verbasizer. It basically took literary source material, randomized it and reordered it to create interesting combinations of words and phrases that Bowie could use as lyrics, or as inspiration for them.
Don’t fool yourself into thinking this makes Bowie any less original. It still takes an extraordinary amount of human intelligence to work these phrases into compelling musical compositions.
If anything, his use of AI to achieve something very human is a lesson for content creators, one that says a lot about today’s creation process. Firstly, we have a lot of data available to us, and we should use it to help us decide what content to create.
We have a lot of data available to us, and we should use it to help us decide what content to create.
For example, software like MarketMuse uses machine learning and AI to analyze top-performing content for certain keywords. It can provide a detailed list of topics that were discussed in well-ranking articles as well as specific questions that were answered. You can then use this as a template to create something new and original.
This article is an example. MarketMuse did not recommend that I talk about Heliograf, InspiroBot or David Bowie for the keyword “machine learning content creation,” but they work as original explainers for concepts that were listed in a brief.
We’re using computer-generated insights to create (hopefully) engaging content for a specific target audience: you.
And that’s just one method of using advanced analytics for content creation. Another might be using free tools like Answer the Public. Just type in any keyword, and it will show you real questions and trending topics that real human searchers have asked of Google. You can use this information to generate new content ideas.
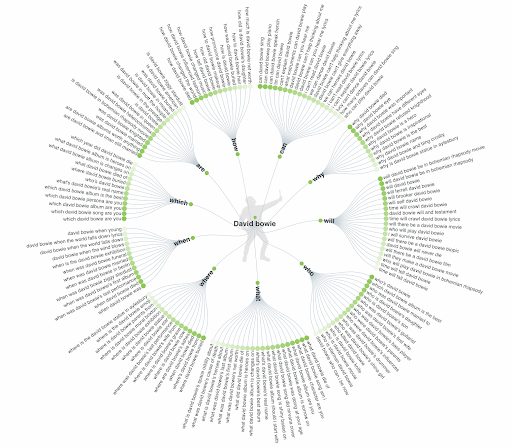
That something extra—creativity, authenticity, original thought, meaning—that helps you connect with your target audience still needs to come from you, though.
*Note that the cut-up technique is believed to have originated with the Dadaist art movement and was championed by poet William S. Boroughs.
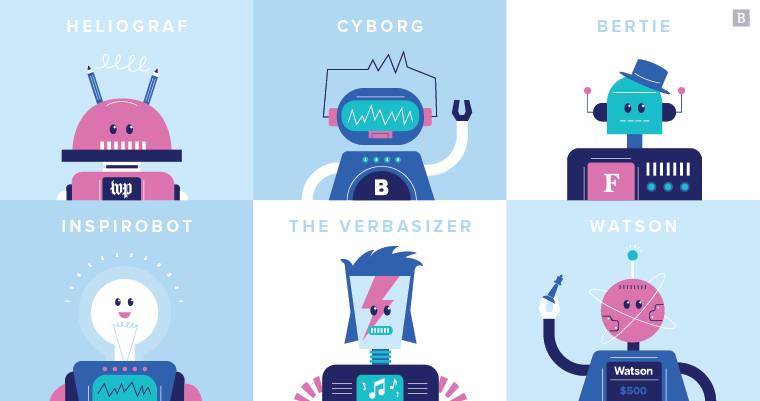
You’re still the Ziggy Stardust of your content creation efforts
Machine learning has changed how we create content. In some cases (a la reporting earnings and high-school sports scores), it even creates the content for us. It can also be valuable in marketing automation efforts, such as segmenting email lists, ad placement and personalization, the development of chatbots that can tap into existing content (e.g., from your FAQs) to answer questions, making timely sales interventions and much more.
But the line is drawn at replicating humans’ ability to espouse the qualitative values that differentiate your brand. So much of marketing is using language to distinguish two products that may actually be incredibly similar in price and function. Even the most savvy marketers have to dig deep to find ways to tell a compelling story that makes their brand more interesting than the competition.
So much of marketing is using language to distinguish two products that may actually be incredibly similar in price and function. Even the most savvy marketers have to dig deep to find ways to tell a compelling story that makes their brand more interesting than the competition.
To understand why bots struggle with these types of endeavors, we need to look at some of the technologies that go into automated content creation.
Natural language processing (NLP)
The simplest way to think of NLP is a computer’s ability to understand written and spoken human language, as opposed to traditional code. Something as basic to you and me as figuring out pronouns is complex for a machine. Homophones and words with two meanings are also challenging.
Still, computers have gotten much better at classifying the function of specific words in certain contexts in order to more fully understand language. We can see this with chatbots, voice search and digital assistants. NLP is also being used for machine translation, which requires an even more precise understanding of language than, say, asking Siri to set a timer.
Natural language generation (NLG)
If the purpose of NLP is to associate different representations of an idea to a singular concept (e.g., knowing that “mow the lawn” and “cut the grass” mean the same thing), natural language generation does the exact opposite: Its job is to find the best of many possible ways to represent a known idea.
NLG is most frequently used in customer support, although it can also be used in content creation. Heliograf is an example. NLG is very much in its early days. Usually, NLG takes simple inputs of information and data and then represents it in a simple manner.
Understanding their limitations in a marketing strategy
NLP and NLG are improving all the time. In 2016, a Japanese-made AI program wrote a novel that passed the first stage of screening for a literary award.
However, even though the scientists who worked on the program called the story a “well-structured-novel,” they admitted that the character development was very weak. This is because non-sentient scribes struggle to relate to the complexities of being human (even if they can string together a logical or believable sequence of events).
Non-sentient scribes struggle to relate to the complexities of being human.
Getting a bot to understand language is very different than endowing it with the ability to say just the right thing at just the right time to elicit a response. That’s the domain of the novelist, the film maker, the visual artist, the musician and the content creator.
So where does machine learning belong in a content strategy?
Data and analytics are hugely helpful in identifying a target audience and in personalizing content for different personas based on performance of those assets and what your users show interest in.
Software-as-a-service (SaaS) offerings including HubSpot’s Content Strategy Tool, Bright Edge, MarketMuse and many others already leverage AI and provide predictive intelligence to help marketers develop keyword- and topic-optimized content that accurately aligns with the searcher intent of very specific personas.
Meanwhile, Azure, AWS, Google and other tech giants are cracking open the Machine learning as a service (MLaaS) market, which will help marketers and software developers harness advanced analytics in new and exciting ways—from developing more advanced chatbots, to automatically generating content meta-tags, making image and media libraries more searchable, recommending social media-based content distribution strategies using real-time data and so much more.
In the not-too-distant future, marketers will even use machine learning algorithms to create ever-better transactional emails, or post basic product updates to a company’s news site or Twitter feed. The New York Times actually developed a quiz that challenges readers to guess what was written by a robot and what was written by a human (I got 50 percent correct).
Despite all of this, robots are far away from attaining the powers of perception needed to repeatedly relate to an audience on a human level. Machines can render a detailed portrait of user behavior on the web, content consumption habits, search queries, word count and so much more. They can even assemble basic sentences using structured data.
Heck, IBM’s Watson beat a master chess player and won at Jeopardy.
But even with their computational power and information, they cannot create a multi-sensory brand experience. They cannot empathize through text or in video with different personas. They do not have imaginations, as they are bound to what they can quantifiably observe.
Maybe that will all change someday. But for now, you’re still the bard. Here are some robo-generated inspirational quotes to remind you as much:


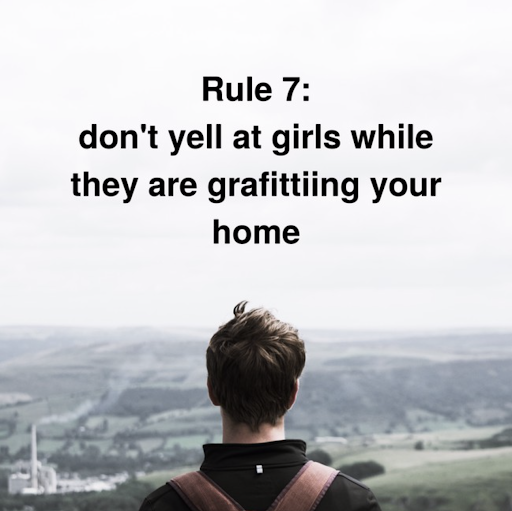

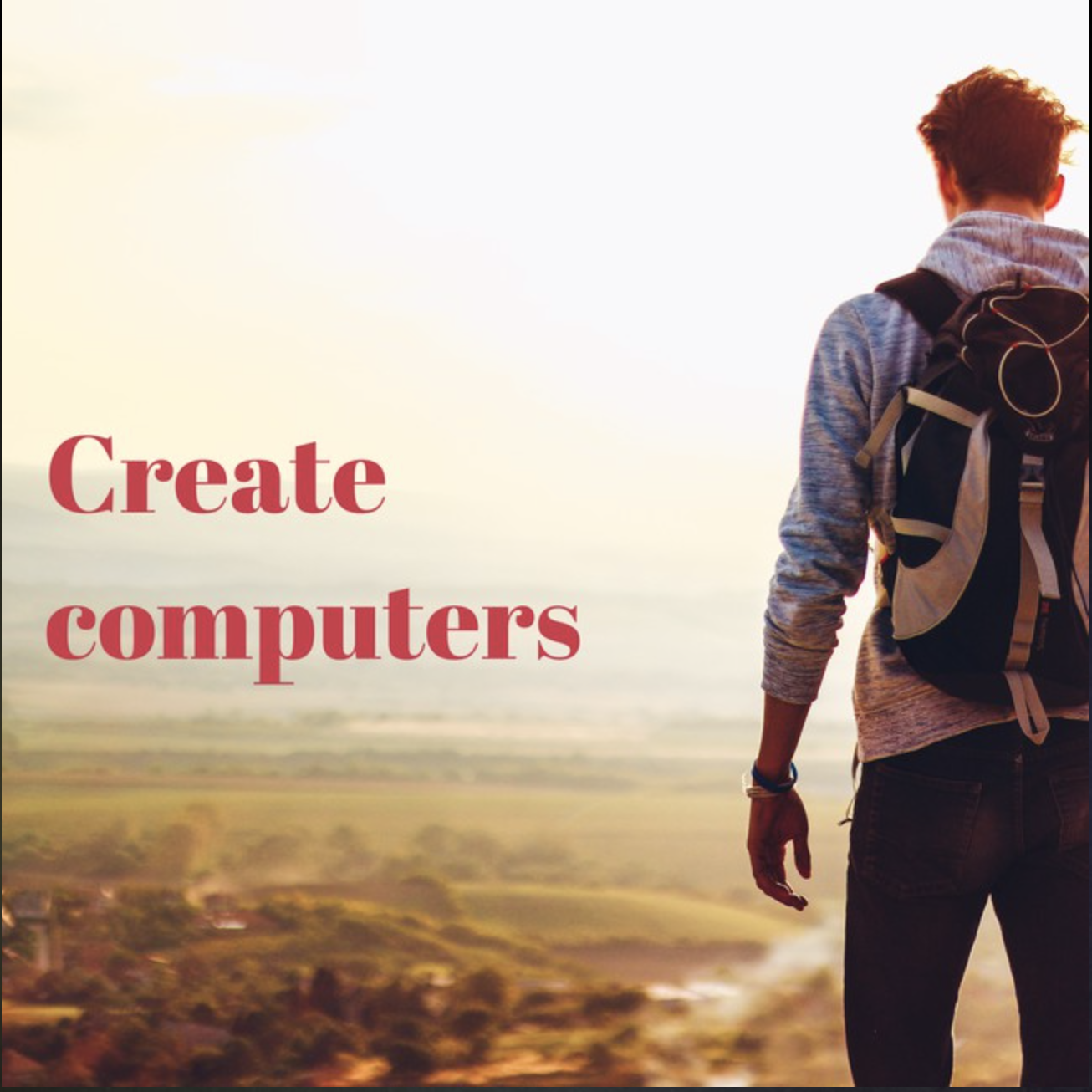
Uh oh.